What is data processing?
Most organisations will process data in some way at some time, but what does the term ‘data processing’ mean? In essence, it is the collection and manipulation of data to produce new relevant information.
It can also include the collection, recording, organisation, structuring, storage, adaptation or alteration, retrieval, consultation, use, disclosure by transmission, dissemination or otherwise making available, alignment or combination, restriction, erasure or destruction of personal data.
Any changes to data can be considered as data processing. Raw data isn’t in the right state for reporting, analytics, business intelligence, machine learning, so it needs to be aggregated, enriched, transformed, filtered, and cleaned.
Data processing certainly isn’t a novel concept, but near-constant technology and software updates could leave anyone’s head spinning. More technology means more data, which makes data processing even more important. Read on to see why data processing should matter to you and your business.
GDPR definition of data processing
Since GDPR came into force in May 2018, there’s an important definition of data processing.
According to Article 4.2 of the EU’s GDPR, processing means “any information relating to an identified or identifiable natural person (‘data subject’); an identifiable natural person is one who can be identified, directly or indirectly, in particular by reference to an identifier such as a name, an identification number, location data, an online identifier or to one or more factors specific to the physical, physiological, genetic, mental, economic, cultural or social identity of that natural person”.
The stages of data processing
Data processing comprises a number of steps that are pretty uniform, however much data you’re hoping to process or what you’re doing with it.
Data collection: Before any processing takes place, the data needs to be collected. Many data collection methods rely on automatic harvesting, but some will be more overt and rely on interactions with data subjects. Whatever means is used to collect the data, it’s essential that it is stored in a format and order that is appropriate to the needs of the business, and that can be easily sourced for processing.
Preparation: Once the data is collected, preliminary work is required to prepare the data for in-depth analysis. For example, this may require a business to only select the data that is required for a particular task, and discarding anything that is incomplete or irrelevant. This typically drastically reduces the time needed to fully process the data, and reduces the likelihood of errors further down the line.
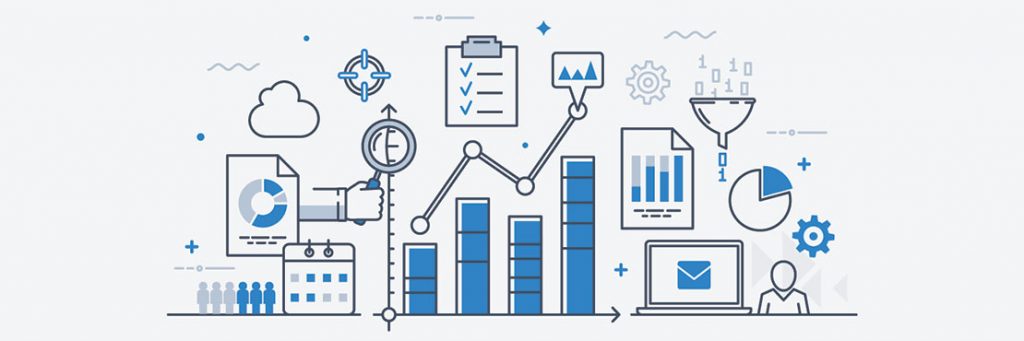
Input: Now that the data has been prepared, what survived the initial filter will be converted into a machine-readable format, one that is supported by the software that will analyse it. The conversion at this stage can be incredibly time-consuming, as the entire data set will need to be double-checked for errors as it is submitted. Any missing or corrupted data at this stage can nullify the results.
Processing: Once submitted, the data is analysed by prebuilt algorithms that manipulate it into a more meaningful format, one that businesses can start to glean information from.
Output: The resulting information can then be manipulated once more into a format suitable for end-users, such as graphs, charts, reports, video and audio, whichever is most suitable for the task. This simplifies the processed data so that businesses can use it to inform their decisions.
Storage: The final stage involves safely storing the data and metadata (data about data) for further use. It should be possible to quickly access stored data as and when required. It’s important that all stored data is kept secure to ensure its integrity.
While each stage is compulsory, the processing element is cyclical, meaning that the output and stage steps can lead to a repeat of the data collection step, starting a new cycle of data processing.
The future of data processing
The future of data processing lies in the last step of the process – storage. Data needs to be quickly and easily accessible, so many businesses are looking toward the cloud. Cloud technology improves upon the current electronic data processing methods, thus making it faster and more efficient.
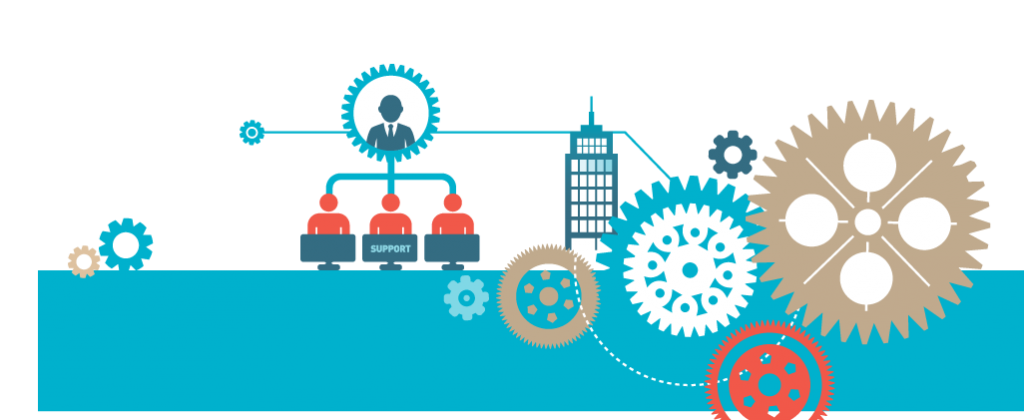
The cloud is especially beneficial for big data – that is, data that is too large for traditional data processing. As IoT and mobile devices surge in popularity, it’s also become synonymous with gathering, analyzing, and using huge amounts of digital information for business operations.
People are producing more and more data, and datasets are becoming larger every day. Because of this, the cloud is the natural next step for data processing. The cloud’s most important selling point is perhaps its inherent adaptability. Technology is constantly evolving and updating, so data processing systems need to be able to adapt quickly. The cloud can easily incorporate software updates and allows companies to combine all of their platforms into one system.
While the cloud is already being used by major big data corporations, smaller companies can benefit from using the cloud as well. Because of their flexibility, cloud platforms provide smaller companies with an opportunity for growth. They can also be quite inexpensive, so cost is not necessarily an obstacle.